Dear curious mind,
I am thrilled to share the latest developments in the world of AI. It's truly exciting to see how open-source AI models are becoming increasingly capable of solving a wide variety of tasks. While cloud-based models from major tech companies continue to grab headlines with their impressive capabilities, I find it particularly fascinating to watch the development of powerful alternatives that can run locally on your own devices.
In this issue:
💡 Shared Insight
Drowning in AI News & My Shift to Privacy-friendly on-device AI
📰 AI Update
Open-Source AI Reaches New Heights: Updated DeepSeek V3 Redefines Non-Reasoning AI
🌟 Media Recommendation
Podcast: Strategic AI Integration to Build More with Smaller Teams
💡 Shared Insight
Drowning in AI News & My Shift to Privacy-friendly on-device AI
It feels impossible to keep up with the pace of innovation in the AI space. Every second week, I host an X audio space and find myself deep in discussion with others, trying to make sense of it all. (Feel free to join us as we are always happy to have new voices and listeners - just go on odd weeks at 9 pm CET to @aidfulai to join).
Recently, just before our latest discussion, there were two major releases from big players in AI. OpenAI integrated image generation directly into their model. The images shared online of this new model are truly stunning (be aware that this model is currently inaccessible in Europe without a VPN). The second big news item was Google’s Gemini 2.5 Pro experimental model rising to the top of the Chatbot Arena. What I appreciate about this ranking (and why I trust it) is the methodology – it’s judged by users choosing the better of two generated answers without knowing the model which created the response.
All of this is incredibly impressive, but my personal focus is shifting. While I acknowledge the power of these cloud-based giants, I am increasingly interested in running models locally on my own computer. This is driven by a desire for privacy – I want to be able to use AI with my personal data without sending it to a cloud provider.
And honestly, what’s happening in the open-source AI world is just as – if not more – exciting than the news from the big cloud AI providers. We’re now seeing open-source models that can run quite fast on a consumer GPU with 24 GB of memory or a MacBook with an M-chip and achieve great results. I am actively experimenting with them for quite some time and realized that we have now achieved a state at which these models can solve many of my daily tasks.
I’m embarking on a personal challenge: I will try to reduce my reliance on ChatGPT, Claude and Grok – my go-to cloud AI providers – and replace them with these powerful local models on my PC. It is an experiment, but I'm excited to explore what's possible and regain control of my data in the process. I will be documenting my progress and sharing what I learn, as I believe the future of AI will be shaped by a combination of cloud models and models running locally on our own devices.
📰 AI Update
Open-Source AI Reaches New Heights: Updated DeepSeek V3 Redefines Non-Reasoning AI DeepSeek News
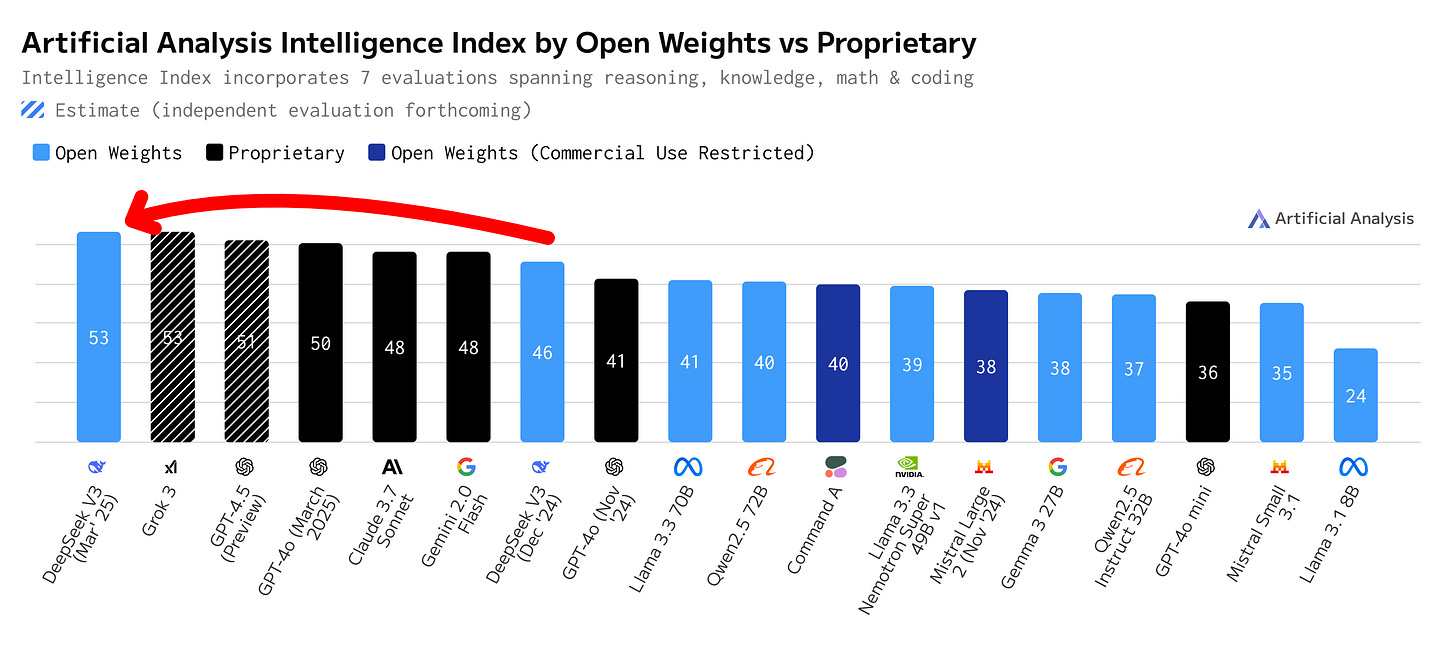
The updated version DeepSeek V3 0324 of the Chinese open-weight model has achieved state-of-the-art performance as a non-reasoning model, according to the AI benchmarking company Artificial Analysis. The model currently outperforms all other open and closed-source models in evaluations covering reasoning, knowledge, math, and coding – a significant step for an openly released model. This is especially great as non-reasoning models produce way fewer words to answer your request, and with that are a lot faster in giving you a response. As the model weights are openly shared, the model can be hosted by anyone and there are already many alternatives to the original app, website and API. You can currently even use it for free via OpenRouter.
🌟 Media Recommendation
Podcast: Strategic AI Integration to Build More with Smaller Teams
A recent episode from the Unicorn Bakery podcast goes deep into the evolving landscape of company building with AI, particularly for startups. The central theme revolves around the surprising efficiency of small teams, leveraging AI-powered tools and streamlined workflows to generate substantial revenue — even reaching tens or hundreds of millions of annual revenue. The episode challenges conventional wisdom about scaling, suggesting that the "throw people at the problem" approach is outdated.
The podcast is in German, and with that not directly accessible by many readers of this newsletter. But the content is so great that I want to share the key aspects in the following with you. If you like to dive deeper, you can chat in the podcast player app Snipd with the episode in English or any other language. Alternatively, you may translate the podcast transcript with an AI tool or even create an English podcast with a tool like ElevenLabs from the original transcript.
Key Aspects
The Power of Small, AI-Enabled Teams: The podcast episode presents compelling examples of companies achieving multi-million dollar annual recurring revenue (ARR) with surprisingly small teams (e.g., Tally reaching $2M ARR with five people). This isn't just about fewer employees; it's about dramatically increased individual productivity through the strategic use of AI tools. The episode emphasizes that the goal isn't necessarily downsizing, but rather empowering existing employees to achieve more with AI assistance. Resistance to change is acknowledged, but the potential for significant revenue increases with the same team size is highlighted.
AI Integration as a Core Strategy: The episode isn't about simply adopting any AI tool; it's about integrating AI strategically into every aspect of the business. This includes AI-driven lead generation, marketing automation, sales processes, and even internal tool development. Several specific tools are mentioned, categorized by function (lead generation, outbound/inbound marketing, lead nurturing, sales conversion, etc.), along with examples of how they are used in different company contexts (e.g., Temple's custom tool built with Cursor and Claude for targeted artist outreach). This demonstrates a shift from hiring more people to solve problems towards leveraging software solutions and AI to increase efficiency.
Marketing and Sales: The episode argues that traditional, broad-based marketing tactics (like large-scale paid social media campaigns) are less effective for smaller companies. Instead, it emphasizes the importance of authentic storytelling, personalized content, and targeted outreach. The CEO often takes on the role of Chief Marketer, building a strong personal brand and engaging directly with potential customers.
Substantive Growth Over Rapid Scaling: The episode cautions against prioritizing rapid growth at the expense of building a solid foundation. A statistic is cited showing that a significant percentage of companies that achieved unicorn status in 2021 subsequently failed to secure further funding, highlighting the importance of sustainable growth strategies. The episode states that focusing on efficient processes and AI-driven tools can mitigate this risk.
In essence, the podcast episode advocates for a new paradigm of company building, emphasising efficiency, AI integration, and sustainable growth over rapid, unsustainable scaling. It provides a compelling case for how small teams, armed with the right tools and strategies, can achieve remarkable success.
Disclaimer: This newsletter is written with the aid of AI. I use AI as an assistant to generate and optimize the text. However, the amount of AI used varies depending on the topic and the content. I always curate and edit the text myself to ensure quality and accuracy. The opinions and views expressed in this newsletter are my own and do not necessarily reflect those of the sources or the AI models.
Another great post Daniel, looking forward to seeing your progress. Would be great to see how an individual could actually start with a local model if your experiment stretches that far. What your selection criteria might be and how you even kick a local model off.