Dear curious mind,
I'm just back from the PKM Summit 2025 in Utrecht, where I had the privilege of connecting with personal knowledge management enthusiasts and thought leaders. The experience was truly transformative, and I'm excited to share some insights I gathered there.
Meanwhile, the AI landscape this week has been dominated by Google's impressive announcements, but so far, not everything works for me as intended.
In this issue:
π‘ Shared Insight
The Two-Way Street: How PKM and AI Will Transform Each Other
π° AI Update
Gemma 3: Google's Compact Open-Weight Models With an Outstanding Performance
The Google Ecosystem Finally Comes Together with Gemini 2.0
NotebookLM Upgrade: Smarter Answers & Citation Preservation
π Media Recommendation
The One-Takeaway Method: Snipd CEO Reveals His Secret to Podcast Learning
π‘ Shared Insight
The Two-Way Street: How PKM and AI Will Transform Each Other
I recently participated in the PKM Summit 2025 held in Utrecht, Netherlands. It was my first time at this gathering of personal knowledge management (PKM) enthusiasts. The event was truly transformative, bringing together thought leaders and practitioners passionate about better ways to capture, organize, and utilize personal knowledge.
The summit experience extended far beyond the formal sessions. Talking in person to people I previously knew only through digital interactions was wonderful. Meeting new people in the hallways and during meals often turned out to be just as interesting.
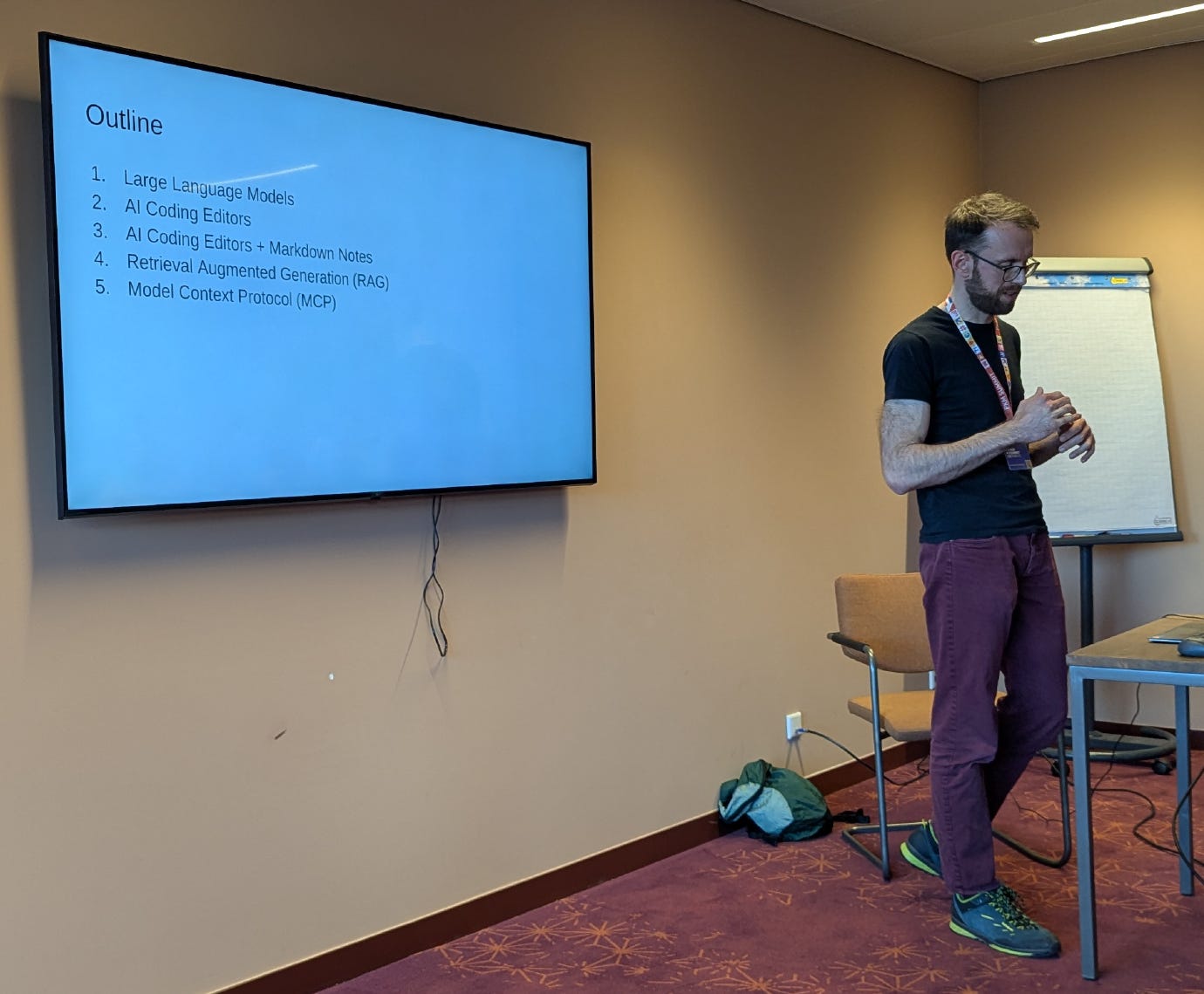
While AI was only one recurring aspect of the diverse program, it naturally captured my attention given my focus in this area. Several speakers touched on AI applications - from automated knowledge capture to the challenges of cognitive offloading in an AI-powered world. Even in sessions not explicitly about technology, the potential for AI integration emerged in audience questions.
The missing AI perspective in PKM discussions
Throughout these conversations, I noticed most discussions centered on how AI will transform our PKM practices. This direction is certainly valuable, but I believe there's an equally important inverse relationship that received surprisingly little attention: Your PKM will fundamentally shape your AI.
This perspective represents a paradigm shift in how we think about both PKM and AI. While we often focus on using AI to enhance our knowledge systems, we should also recognize the value our personal knowledge repositories hold for being used by personalized AI assistants. The notes, connections, and insights captured in a well-maintained PKM system represent a unique dataset containing:
Your specific vocabulary and conceptual frameworks
The connections you've made between ideas
Your professional and personal contexts
The questions that matter most to you
This treasure trove of personal data is the perfect foundation for creating an AI assistant that truly understands your thinking patterns and preferences. While general-purpose AI assistants must serve millions of users with diverse needs, an AI accessing your personal knowledge could adapt specifically to your knowledge and interests.
In essence, the hours you spend building and refining your PKM system aren't just helping you organize information today - they're creating the foundation for a deeply personalized AI assistant tomorrow. Your notes become more than just external memory; they become the training ground for an AI that thinks like you. As AI continues to evolve, those who have invested in thoughtful PKM systems will likely have a significant advantage. Their personal datasets will enable AI assistants that don't just retrieve information, but truly understand their unique cognitive patterns and needs.
The future of PKM isn't just about how we will use AI - it's about how our carefully cultivated knowledge will shape the AI that serves us. This insight may not have been the focus of the summit discussions, but I think it represents one of the most exciting possibilities at the intersection of PKM and AI.
π° AI Update
Gemma 3: Google's Compact Open-Weight Models With an Outstanding Performance [Google blog]
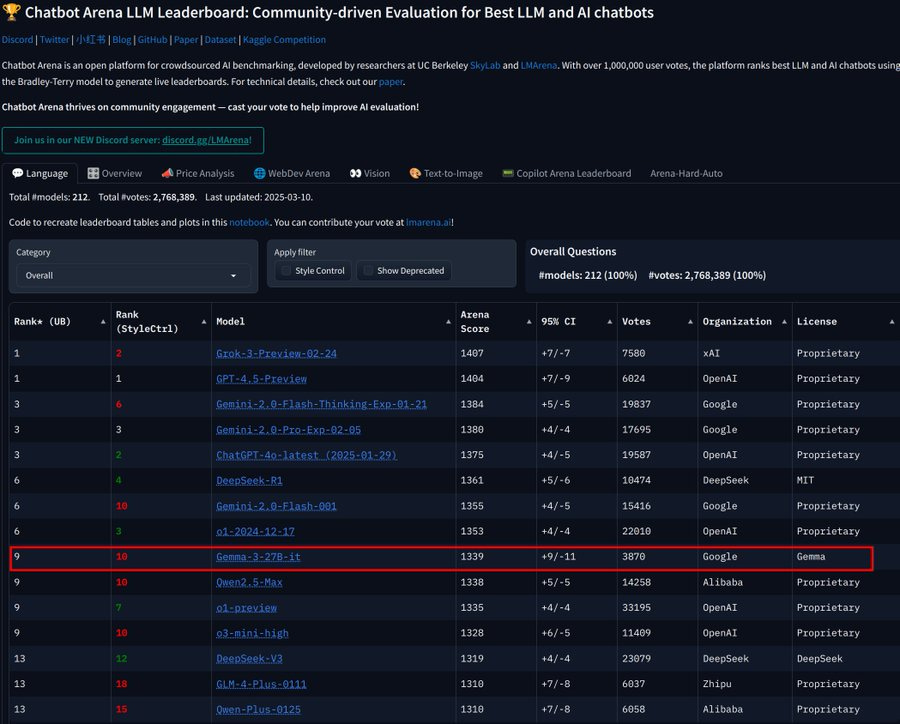
Google has released Gemma 3, an impressive open-weight model featuring a 128K context window, image understanding capabilities, and support for over 140 languages. The model is available in four sizes (1B, 4B, 12B, and 27B parameters). You can test it in Google AI Studio or run it locally on your own hardware through platforms like Ollama.
Googleβs Cloud-based Chatbot Gemini Gets Personal [Google blog]
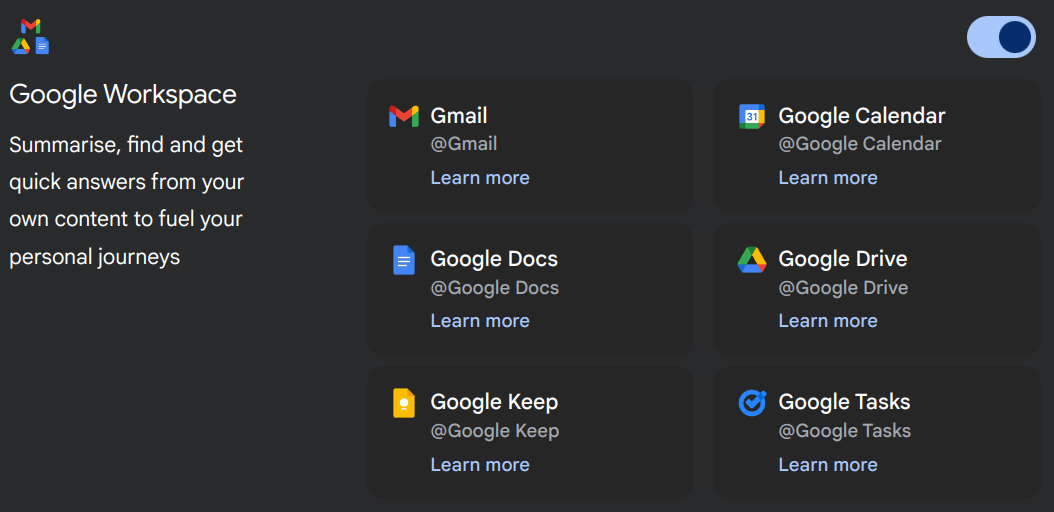
Google's latest Gemini update now leverages your search history and integrates with more Google tools, creating a truly personalized AI assistant that understands your preferences and past interactions. A great update if your digital life is centered around the offerings from Google. However, so far, the βPersonalization (experimental)β model does not show for me, and likely all other EI users, in the model drop-down menu.
NotebookLM Upgrade: Smarter Answers & Citation Preservation [π post by Josh Woodward, Google Labs VP]
The latest version of NotebookLM uses Gemini 2.0 Flash Thinking for better responses. Furthermore, by preserving citations when saving replies to notes, my biggest pain point was solved. At least in theory, as it does not work as intended on my Linux system.
π Media Recommendation
The One-Takeaway Method: Snipd CEO Reveals His Secret to Podcast Learning
The Snipd podcast player is one of my favorite apps, and I recommended it already multiple times before in this newsletter.
In a recent Latent Space podcast episode, Snipd CEO Kevin Smith explained how the app helps listeners capture and process insights from podcasts through several key AI features:
Instant capture: Use the back shortcut on your headphones to save and summarize important moments
Smart transcription: Automatically identifies speakers, timestamps, and even book recommendations
Interactive learning: Chat with podcasts to find specific information or get personalized summaries
This podcast episode provides insights into Snipd's development, revealing how they tackle technical challenges like speaker diarization (separating different speakers in an audio recording), implement "LLM as judge" techniques to improve accuracy, and strategically balance AI model costs with performance.
Listeners get a glimpse of Snipd's future direction, including plans for voice-based AI companions that engage users after episodes end, expansion into video podcasts and more personalized content discovery experiences.
My take: I love the practice shared by Kevin: Take time after listening to a podcast episode to identify just one key takeaway from all you've heard. This simple habit serves as a "forcing function" that helps you process information more deeply and significantly improves knowledge retention. I will try to do this from now on for each podcast episode I listen to. On top, I already applied this to all the talks I attended on the PKM Summit 2025, and it feels transformational.
Disclaimer: This newsletter is written with the aid of AI. I use AI as an assistant to generate and optimize the text. However, the amount of AI used varies depending on the topic and the content. I always curate and edit the text myself to ensure quality and accuracy. The opinions and views expressed in this newsletter are my own and do not necessarily reflect those of the sources or the AI models.